phd work
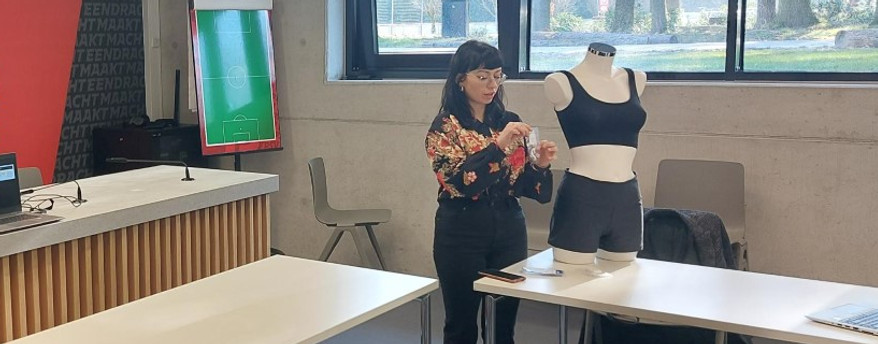
Year 1
I took time to explore and investigate in depth one of the use cases - patient remote monitoring in cardiac rehabiliation. The sensors which are used in remote monitoring gather data such as ECG, acceleration, heart rate variability, body temperature. Most solutions for self-management are very tech-centric and within these systems, patients become merely a 'source of data'. I wanted to understand what other aspects that could be eventually turned into data we can track, are relevant for a cardiac patient. Ultimately, I also explored what solutions domain experts (clinicians, cardiac rehabilitation epxerts) can envision based on machine learning (ML) heuristics. I did this through the following activities:
-
An interview study with 7 domain experts to explore the cardiac rehabilitation patient journey in relation to the clinician based on a list of influencing factors as a starting point for the conversation. Participants were presented with a list of influencing factors of patient behavior in cardiac rehabilitation. This list consists of three categories of influencing factors for attendance, adherence, completion of the cardiac rehabilitation, as well as managing one’s health. The three umbrella categories were:
-
sociodemographic
-
centre-related and practicalities
-
psychological
-
cognitive and personality-related
-
and clinical
-
These categories were based on early and recent work which focuses on unpacking the complexity of a cardiac patient’s journey through rehabilitation and after. The overview of factors is a tool meant to start the conversation around which influencing factors are more relevant to the behavior the participants’ expertise gravitates around (e.g., dietary intake for a nutritionist). Participants were also asked to give insights into what factors are missing from the framework, and the various interconnections between the factors (e.g., older patients - sociodemographic factor - may have difficulty in travelling to the cardiac rehabilitation center - centre-related factor), based on their own expertise.
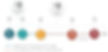
2. A workshop with 6 domain experts to identify key problem spaces and envision solutions with the help of the ML capabilities. After the general pain points and frictions of the cardiac rehab patient journey were described, participants were then asked to identify certain solutions or preferred scenarios using or starting from the list of ML capabilities.
Participants were explained that the given verbs refer to abilities provided by ML technologies. However, because the knowledge about ML varied significantly from participant to participant, and most participants have a clinical and not a technical background, we did not intend for them to propose technological solutions. Our aim was to observe whether ideas could be generated starting from our abstractions of ML capabilities. Therefore, they were asked to envision ideal scenarios where, with the help of different tools, they could be able to do anything they would like to do when it comes to their practice.
Year 2
Remote monitoring of patients undergoing prehabilitation or rehabilitation to monitor their physical activity behavior calls for contextual data gathering schedule and activity labelling, motivation/reason for increased or decreased activity.
Design of ecological momentary assessment for physical activity monitoring of patients in the wild:
-
dealing engagement and habituation of users, validity of data
-
dealing with limited adoption and resistance from clinical researchers
-
dealing with different modes of use: EMA technologies tailored to the individual/group of individuals (e.g. test one text based, one digital visual, one tangible)
Other emerging questions: How can we represent this data for clinicians and leverage it within their practice and how can it change the clinician-patient dynamic?